AI in Supply Chain Management for Distributors
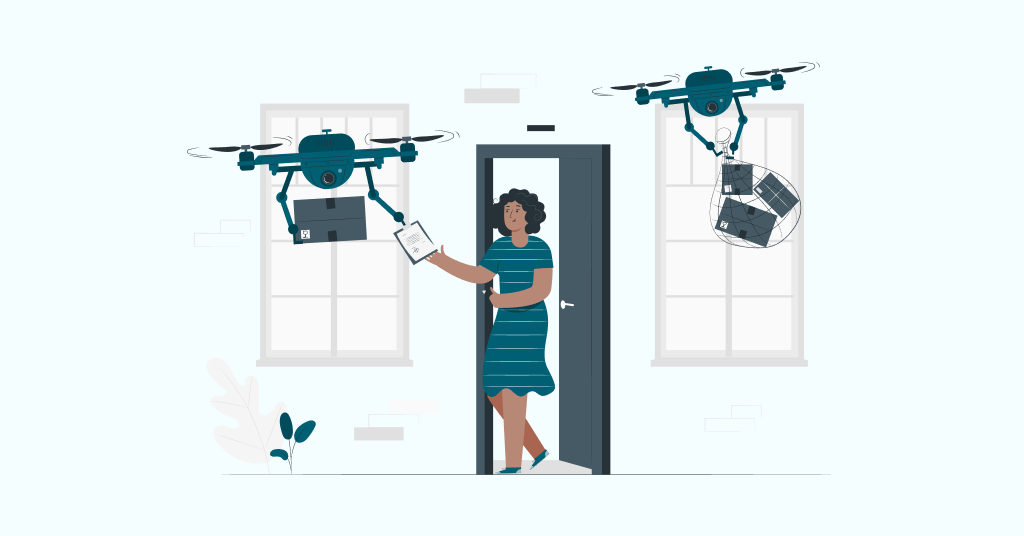
Artificial Intelligence (AI) is today’s most important change in supply chain management. Distributors have access to the newest technologies that improve efficiency and the quality of decision-making. The AI in the supply chain market? It will surge from USD 730.6 million in 2018 to an incredible USD 10,110.2 million by 2025, with a fantastic CAGR of 45.55%. B2B distributor today see an explosion in the demand for AI, which signifies the urgency for integrating AI technology.
AI technology allows companies to automate most parts of their processes, ensure forecasting accuracy, and bring costs to a minimum level. It is an essential factor for businesses to remain competitive. This article will look at AI’s influence on supply chain management. Let’s start by looking at this AI-driven future in supply chain management.
What is AI in Supply Chain Management?
AI in supply chain management refers to artificial intelligence technologies, such as machine learning, data analytics, and automation, that improve and optimize supply chain processes. This incorporates demand forecasting, stock management, logistics, procurement, and supplier relationship management.
AI can spot trends and forecast market directions by examining much data. It saves time and energy by automating routine tasks and increasing accuracy and business efficiency. For distributors, AI permits immediate feedback and more efficient operations, enabling them to react promptly to demand changes, smooth operational flows, and save costs.
Benefits of AI in Supply Chain Management for Distributors
AI in supply chain management boosts efficiency, cuts costs, and promotes sustainability. B2B platform can transform their supply chains into agile, data-driven frameworks using AI.
Improved Demand Forecasting
AI tools analyze vast historical data, market conditions, and external factors like weather. Traditional methods rely on historical data and manual estimates and are prone to inaccuracies. AI identifies patterns in real-time, enabling precise demand forecasts. Businesses can avoid overstocking or stockouts, ensuring optimal product availability. This improves efficiency and customer satisfaction.
Enhanced Efficiency
AI automates repetitive tasks like order processing, inventory updates, and invoice management. This reduces human error and frees employees from strategic activities like market analysis and supplier optimization. With AI handling routine tasks, supply chains become faster and more responsive.
Cost Reduction
AI optimizes supply chain elements, leading to significant savings. Accurate demand forecasting reduces excess inventory, lowering storage costs. In logistics, AI analyzes traffic and weather to optimize routes, cutting transportation expenses. Automation reduces labor costs for repetitive tasks, and predictive analytics prevent costly disruptions.
Real-Time Data and Insights
AI provides real-time visibility across the supply chain. Traditional methods rely on periodic reports, delaying decisions. AI constantly monitors data, enabling quick, accurate adjustments. If a supplier delays, AI suggests alternatives or reroutes shipments, maintaining efficiency.
Predictive Maintenance
AI monitors equipment like automobiles and warehouse machinery in real time. It analyzes sensor data to predict maintenance needs, preventing unexpected breakdowns. For example, AI detects wear and tear, allowing preemptive maintenance, ensuring continuous operations and avoiding costly delays.
Better Supplier and Risk Management
AI enhances supplier management and risk mitigation. It continuously monitors supplier performance, tracking delivery times and product quality. AI also analyzes global news and weather to predict disruptions. If a natural disaster threatens a supplier, AI alerts the company to find alternatives, mitigating risks.
Optimized Logistics and Transportation
AI optimizes logistics by analyzing traffic patterns, fuel consumption, and driver behavior. It ensures efficient delivery routes, reducing fuel costs and delivery times. AI accounts for real-time conditions like weather disruptions, ensuring on-time deliveries and improving customer satisfaction.
Sustainability and Waste Reduction
AI helps companies meet sustainability goals by optimizing resource use. Accurate demand forecasting reduces overproduction and waste. In logistics, AI minimizes fuel consumption and carbon emissions. AI also helps manage warehouse energy use, supporting sustainability initiatives and improving the bottom line.
Real-World Examples of AI in Supply Chain Management
AI has enabled companies to improve the quality of decision-making and has certainly boosted overall efficiency. The following are some real-life examples of AI that are used in different sections of a supply chain.
Demand Forecasting
AI is changing the game by making use of next-gen technologies like machine learning and predictive analytics in the domain of demand forecasting. For instance, IBM Watson Supply Chain examines market trends, historical data, and customer conduct, which is a perfect example of how AI can correctly predict future demand.
The data processed in real-time can help businesses make smart decisions about the level of stock they should have in store, which keeps them from running out of stock or holding overstock. They can also better understand their storing and production planning operations. This proactive approach reduces product shortages, reserves, and overall supply chain efficiency.
Inventory/Warehouse Automation
One of the key areas where the concept is being implemented is in warehouses. Take DCKAP Integrator as a prime example. This integration and automation solution is tailor-made for distributors. With a team of AI experts and dedicated support, DCKAP Integrator enhances logistics processes. It optimizes inventory levels and automates customer service, making business life easier.
The automation features let companies streamline repetitive tasks such as document routing, data entry, and approval processes. This cuts down on errors and simplifies operations. Businesses save precious time, allowing logistics firms to grow without getting bogged down by manual tasks.
Transportation and Route Optimization
UPS’s ORION (On-Road Integrated Optimization and Navigation) is a good example of a machine learning and data analytics-based transportation system. It is a smart system that utilizes machine learning and data analytics to find the most efficient delivery routes.
This system enables UPS to reduce the number of kilometers driven by optimizing route choice. These benefits are less fuel consumption, faster delivery times, and lower carbon emissions. Moreover, AI-based route optimization is a significant advance in logistics that makes transportation more sustainable and cost-effective.
Predictive Maintenance
AI technologies like the Internet of Things (IoT) and machine learning enable predictive maintenance. For example, the GE Digital Predix platform constantly observes the state of the industrial machinery. It anticipates equipment’s likely failure, which helps proactively schedule maintenance, thus avoiding any breakdowns.
This proactive approach leads to less unexpected downtime, longer equipment life, and decreased maintenance expenses. The issues of reliable operation are resolved by employing AI-driven predictive maintenance. Thus, expensive interruptions are no longer a problem. Also, the device’s reliability is largely improved.
Supply Chain Risk Management
Platforms like Resilinc use machine learning and big data analytics to track global risks. From natural disasters to political turmoil, AI gives early warnings, allowing businesses to take action before disruptions strike. This boosts supply chain resilience, enabling companies to react fast and reduce the fallout from unexpected events.
Challenges of Adopting AI in Supply Chain Management
Adopting AI in supply chain management is transformative. Yet, it brings significant challenges. Organizations must navigate these obstacles for successful implementation.
Data Quality and Integration
AI needs high-quality data for precise predictions. However, supply chain data often comes from multiple sources, like ERP systems, IoT sensors, and third-party providers. Ensuring this data is accurate, complete, and consistent is tough. Inaccurate data can lead to poor AI outputs, undermining its value in the supply chain.
Lack of Skilled Talent
To adopt AI successfully, organizations need skilled teams. These teams must understand data science, supply chain operations, and AI tools. Unfortunately, there’s a shortage of professionals with this blend of skills. Without the right talent, companies struggle to harness AI’s full potential.
Complexity and Scale of Supply Chains
Supply chains are intricate, with many interconnected processes, b2b suppliers, and stakeholders. Creating AI models that capture this complexity is challenging. Additionally, global supply chains operate on a large scale, making it difficult to deploy effective AI solutions across various regions.
Integration with Existing Systems
Many companies rely on legacy systems incompatible with modern AI technologies. Upgrading these systems requires time and significant investment. Integrating AI into existing infrastructure can be complicated and often requires retraining employees.
Interpretability and Trust
AI models, particularly deep learning ones, often seem like “black boxes.” Their decision-making processes lack transparency. This can lead to skepticism among supply chain professionals, who may hesitate to trust AI insights without clear explanations. Improving AI’s interpretability is essential for building trust.
Data Security and Privacy
AI depends on vast amounts of sensitive data, raising security and privacy concerns. Organizations must protect this data from cyber threats and comply with regulations like GDPR and CCPA. Ensuring robust security while managing large data volumes complicates AI adoption.
How to Prepare Your Supply Chain for AI Integration?
Integrating AI into supply chain management can seem challenging and expensive. However, businesses can take key steps to prepare their legacy systems for this upgrade.
Audit Value Creation
Begin with a thorough audit of your logistics network. Identify bottlenecks, productivity drains, and error-prone processes. This review will highlight where AI can add the most value. Knowing where to focus is essential.
Create a Strategy and Roadmap
Modernizing your supply chain is complex. You’ll face many challenges and need to satisfy various leaders. Rather than upgrading everything at once, set priorities. Develop a strategy that addresses urgent issues first. Your roadmap should ensure each project builds on the last and has proper funding.
Design a Solution
Once you’ve pinpointed the area for AI enhancement, start designing your solution. Consider necessary systems like cloud applications, edge servers, and IoT devices. Think about how these will fit with your existing infrastructure. This is often when companies consult with systems integrators or industry experts.
Select a Vendor
Choose your technology vendor carefully. Many offer AI solutions, but not all will meet your needs. Look for a vendor whose capabilities match your requirements. Evaluate their pricing, support, and company culture.
Implementation and Integration
After selecting a vendor, it’s time for implementation. A systems integrator will work with your IT team to install and integrate systems. Expect some downtime and training for employees during this phase. With solid planning, you can make a smooth transition to production.
Remember Change Management
Change can be unsettling for employees who are used to old and inefficient methods. Prepare your team before launching your AI solution. Clearly explain the reasons for the change, the productivity gains expected, and the success metrics you’ll use.
Monitor and Adjust
AI projects are not one-time efforts. These systems need ongoing monitoring and adjustments. Even if everything seems to be running well, experiment with changes and gather data to improve performance further.
Future Potential of AI in Supply Chain Management for Distributors
As AI evolves, it will reshape many aspects of supply chains, boosting efficiency, agility, and resilience. Here are key trends and predictions for AI’s role in this field:
Autonomous Supply Chain Operations
Autonomous supply chains are on the rise. AI and machine learning will handle planning and execution tasks with minimal human input. This shift will automate demand forecasting, inventory control, and logistics management, leading to more efficient operations. Predictions suggest that supply chains will become highly autonomous in the next decade, with AI managing much of the workload.
Predictive and Prescriptive Analytics
AI-driven predictive analytics will improve, allowing distributors to forecast market trends and customer needs accurately. This will enable proactive decisions, like adjusting inventory levels or launching targeted marketing campaigns based on real-time data. Moving towards prescriptive analytics will enhance decision-making by providing actionable recommendations to optimize supply chain performance.
Collaboration Across the Supply Chain
Future AI applications will boost collaboration among supply chain partners using technologies like blockchain and smart contracts. This integration will enhance transparency and trust, allowing real-time tracking of goods and verification of transactions. Improved collaboration will create more resilient supply chains that respond quickly to disruptions.
Human-AI Collaboration
AI will enhance human capabilities, not replace them. By automating routine tasks and providing data insights, AI will allow supply chain professionals to focus on strategic activities. This teamwork will lead to smarter processes and better responsiveness to market changes.
Sustainability Initiatives
AI will be essential in promoting sustainability in supply chains. AI can help reduce waste and lower carbon footprints by optimizing logistics routes and managing inventory. Companies will increasingly use AI tools to monitor sustainability practices, ensuring they comply with environmental regulations while boosting efficiency.
Real-Time Decision Making
Combining AI with edge computing will enable real-time decision-making in supply chains. Distributors will respond quickly to changes, such as demand fluctuations or unexpected disruptions. AI-driven analytics will enhance visibility into operations, helping inform decisions at all supply chain levels.
Conclusion
Artificial Intelligence is changing the game in distributors’ supply chain management. It brings new levels of efficiency, accuracy, and responsiveness. With AI-driven tools, companies can improve demand forecasting, streamline operations, cut costs, and boost customer satisfaction.
Now, integrating AI technologies is essential for staying competitive. However, adopting AI isn’t without its challenges. It requires careful planning and strategic implementation. By using advanced solutions like DCKAP Integrator, you can build a strong and resilient supply chain. Contact us today for more details on how DCKAP Integrator can elevate your business!
Discover the preferred eCommerce platform for distributors and elevate your B2B business to new heights with industry-leading solutions!